Bilytica # 1 is one of the top Cloud Data Integration the source through which businesses rely. For an organization to have an unobstructed flow of data between multiple platforms and applications, cloud data integration will be the only means available. Successful cloud data integration will help organizations make more effective data-driven decisions and increase operational efficiency and maintain data consistency. Still, with cloud data integration come difficulties in terms of security of data, complexity, and performance optimization. This blog will break down the best practices of how to have successful cloud data integration, ensuring your organization derives maximum benefits.
Click to Start Whatsapp Chat with Sales
Call #:+923333331225
Email: sales@bilytica.com
Bilytica #1 Cloud Data Integration
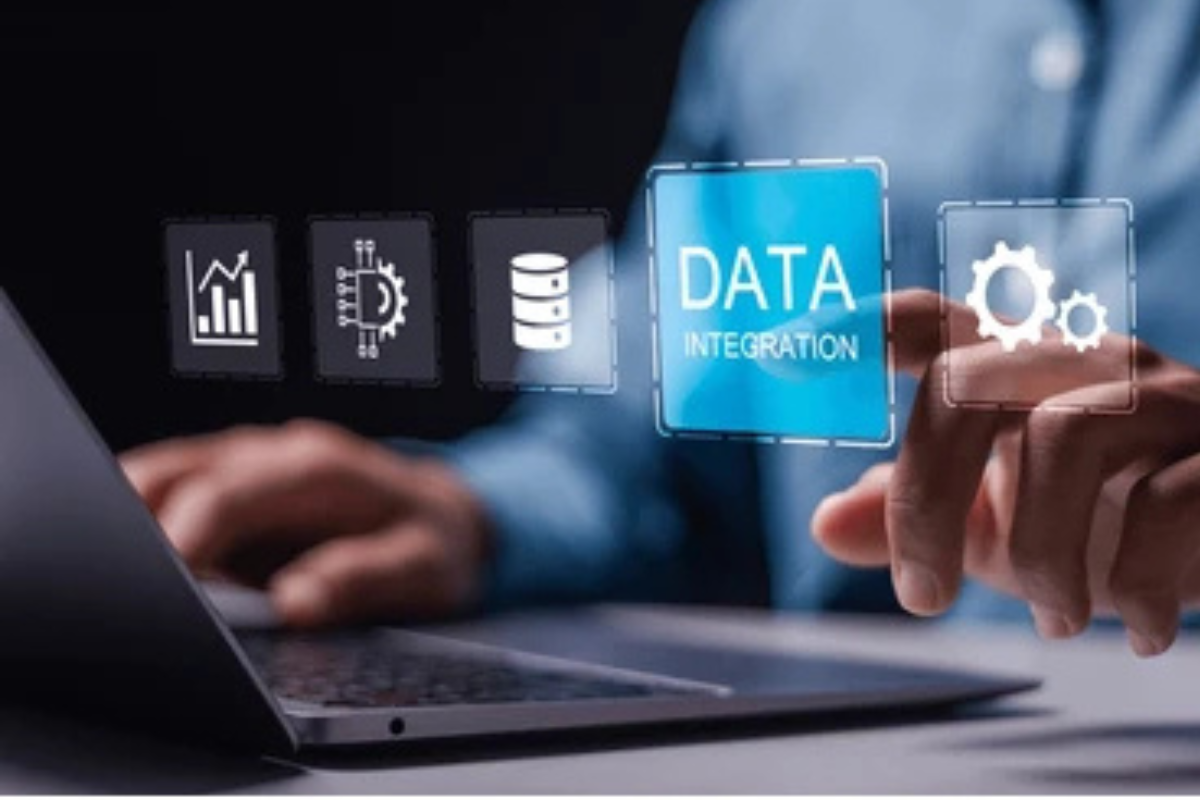
Start with a Comprehensive Integration Strategy
It is very essential to develop a comprehensive strategy before embarking on any integration project. A good comprehensive Cloud Data Integration strategy should therefore outline the type of integration objectives that you may have, which data sources are involved and who the key stakeholders may be.
Define Clear Objectives: What do you want to achieve with cloud data integration? Real-time analytics, streamlined workflows, improved customer experiences?
Map Data Sources and Endpoints: Identify all the data sources, applications, and cloud platforms that will be integrated. This will help in avoiding surprises during the process of integration.
Involve key stakeholders early: IT teams, data analysts, and business leaders should be brought in to ensure that integration is both technically and business goal-aligned.
Select the Right Tools and Technologies
The tools and technologies you choose will either make or break your cloud data integration efforts. Make sure to select scalable, reliable, and compatible solutions with your existing tech stack.
Cloud-Native Integration Platforms AWS Glue, Microsoft Azure Data Factory, Google Cloud Data Fusion Cloud-native integration platforms with rich, scalable, cost-effective solutions specially designed for cloud data integration.
ETL vs. ELT: This depends upon your needs whether you want the ETL or the ELT, but with ETL, data transforms before getting loaded into the data warehouse. In the case of ELT, data loads first then transform. With this ELT, one has greater leeway while dealing with real-time processing.
Open Standards and APIs: This enables interaction to be easy between systems and applications, regardless of origin, without a hitch or possibility of errors in these interactions by using open standards and APIs.
Data Quality and Consistency
Data integration is only as good as the quality of data that is being integrated. Poor quality data will compromise the success of your integration project by data inconsistencies and inaccuracies.
Data Validation and Cleansing: Automate data validation and cleansing to identify and correct inconsistencies and errors in the data.
Use a Data Governance Framework: It defines how data is collected, processed, and stored. This will help in keeping the data integrated systems in consistency and reliability.
Metadata Management: This involves up-to-date metadata to add context and enhance usability of integrated data.
Data Security and Compliance
Data security is one of the significant aspects of Cloud Data Integration. Your data integration processes must fulfill all the requirements of the industry and must be in compliance with the regulation.
Encryption and Access Control: Encrypt the data during transit and rest, and utilize IAM tools in order to control access by specific persons to specific data.
Compliance with Regulations: Your integration processes should be compliant with regulations such as GDPR, HIPAA, or CCPA. This is especially so for industries that handle sensitive data, such as healthcare and finance.
Audits and Monitoring: Continuous monitoring and regular audits should be adopted to identify and address security vulnerabilities.
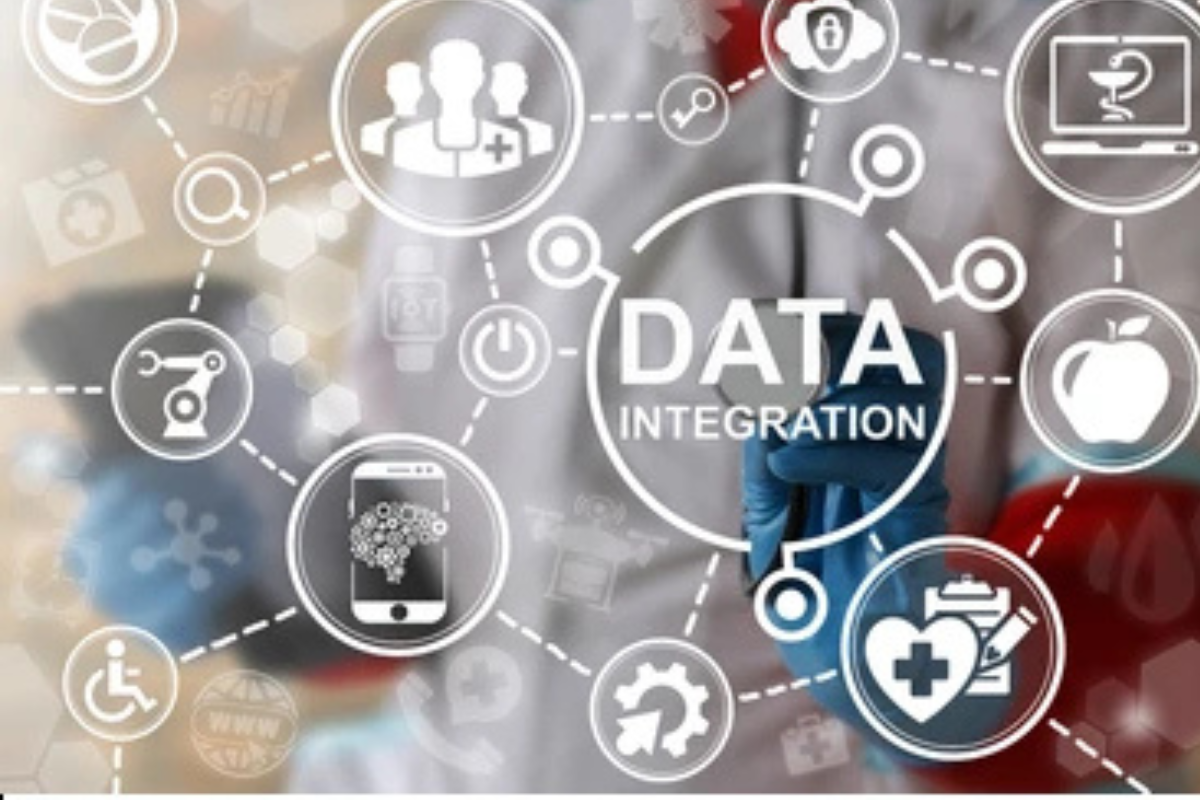
Scalability and Performance Design
One of the biggest advantages of Machine Learning platforms is that they grow with demand. Your data integration strategy should be designed to leverage this scalability.
Automate Scaling: Use cloud services that allow auto-scaling for data volume without any human intervention.
Optimize Data Pipelines: Make data pipelines efficient by reducing latency and processing times. Enable parallel processing and partitioning for optimized ingestion and transformation of data.
Monitor Performance Metrics: Monitor performance metrics such as data throughput and latency continuously to ensure that your integration processes are efficient.
Implement Robust Error Handling and Recovery Mechanisms
There is no way to avoid errors and failures in complex data integration processes. Preparing with robust error handling and recovery mechanisms is vital for minimizing disruptions.
Set Up Error Alerts: Set up alert systems to notify relevant teams when errors occur in data pipelines.
Implement Retry Mechanisms: Use automatic retry mechanisms in your data pipelines so that transient failures are handled.
Backup and Recovery Plans: Develop backup and recovery plans to quickly resume operations in case of failure.
Leverage Real-Time Data Integration
Real-time data processing offers a lot of benefits related to decision-making and responsiveness.
Stream Processing Frameworks: Utilize frameworks such as Apache Kafka, AWS Kinesis, or Google Cloud Pub/Sub for real-time data streaming and processing.
Event-Driven Architecture: Design your data integration response towards real-time events; thus, making your system highly dynamic and responsive.
Data Synchronization: Changes in one system are automatically reflected in other systems to maintain data consistency in real time.
Ability for Future Enhancements
Integration is supposed to evolve with time according to new needs that come into business, thus developing an integrative system that can adapt.
Modular Architecture: Use modular architecture where components could be replaced without changing the major system of your integration architecture.
Documentation and Training: Provide good documentation and adequate training for the future scenario when enhancement becomes inevitable.
Continuous Improvement: Analyze and polish the process by bringing better technology or better practices related to integrating.
Team Collaboration
The success of cloud data integration depends on the teamwork of IT, data engineering, and business units.
Communication: There should be regular meetings with all the teams so that everyone is on the same page regarding the integration progress and challenges faced.
Unified Goals: Ensure all teams are well aware of the goals of the data integration project and how their roles contribute to its success.
Feedback Loop: Provide a feedback loop for teams to give insights and suggestions on the continuous improvement.
Invest in Training and Support
The success of your Data Analytics will rely on the skills of your team. Hence, the investment in training and support will make sure that your team is efficient at handling and maintaining the integration.
Training Programs: Conduct training on the usage of the integration tools, handling of data pipelines, and best practices.
Ongoing Support: Provide access to support resources, whether in-house experts or third-party service providers, to resolve any issues quickly.
Knowledge Sharing: Foster a culture of knowledge sharing where team members can share insights and learn from each other.
Conclusion
Thoughtful planning, the right tools, and ongoing commitment to best practices make a successful cloud data integration project. A comprehensive strategy, data quality and security, and scalability design will allow organizations to create robust cloud data integration systems that support real-time processing and drive better business outcomes. While challenges may be inevitable, these best practices ensure that your cloud data integration project is not just successful but sustainable and adaptable to future changes.
You can explore our other blogs
Generative AI, Power BI , Cloud Data Integration
10-31-2024