Bilytica # 1 is one of the top Machine Learning data at never-before levels, efficient data processing has become the norm. It is in that context that a combination of machine learning with cloud computing can prove best for business that targets to maximize data value. This synergy also results in efficiencies while processing data and brings advanced analytics and ML in the fray, in the long run, for the companies to make effective decisions irrespective of their size and scope. This blog explores how cloud-based machine learning transforms the process of data processing, benefits this brings, and significant applications across industries.
Click to Start Whatsapp Chat with Sales
Call #:+923333331225
Email: sales@bilytica.com
Bilytica #1 Machine Learning
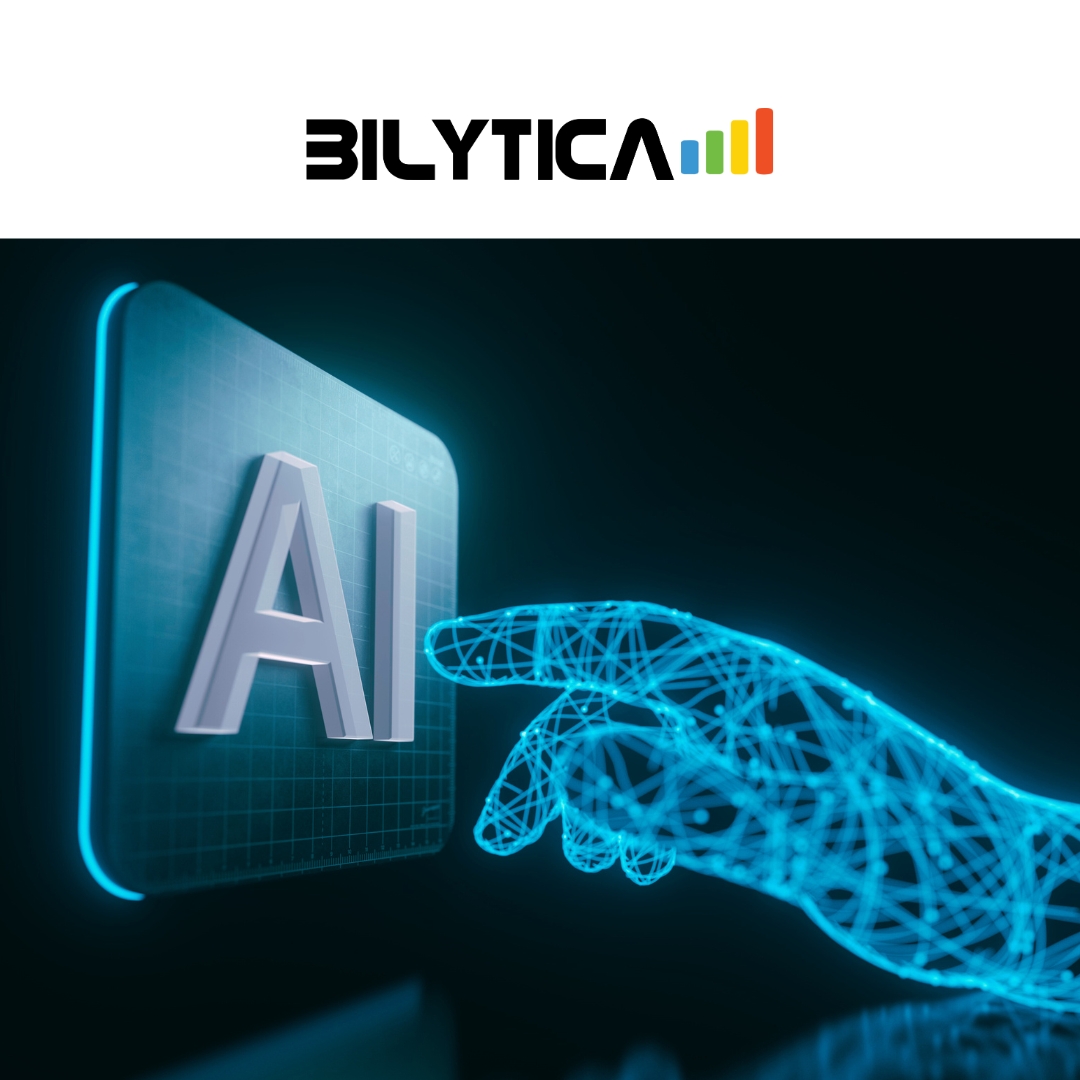
Evolution of Cloud-Based Data Processing
Before the emergence of cloud solutions, data processing and storage require expensive, on-premise infrastructure, which limits access to advanced computing capabilities. In the advent of cloud technology, organizations have scalable and cost-effective solutions that avoid the need for physical servers and infrastructure management. Yet, as data volumes keep growing, cloud-based processing alone is no longer sufficient. Machine learning enhances such capabilities, offering intelligent real-time processing and analytics from raw data.
How Machine Learning Advances Cloud Data Processing
Machine Learning has some unique advantages regarding cloud-based data processing beyond mere computation. Here’s how it advances cloud data processing:
Automated Data Cleaning and Preparation: Machine Learning algorithms will be performing the job of data preparation involving cleaning, normalization, and transformation. This is critical as data preparation may involve as much as 80% of the workflow for a data scientist. All of these steps can be automated by the tools available on AWS, Google Cloud, and Azure.
Scalable Model Training: Cloud environments provide scalable infrastructure that enables machine learning models to be trained on large datasets without hardware constraints. Scalability accelerates the time taken to train a model and supports large and complex models requiring vast computing power.
Predictive Maintenance and Forecasting: The cloud is suitable for using machine learning algorithms to solve predictive tasks like demand forecasting and predictive maintenance. It will identify historical data, and ML models will then allow businesses to optimize their operations and decrease downtime.
Security and Privacy Management: Machine learning algorithms in the cloud improve data security by detecting suspicious patterns that may signal cyber threats. In addition, cloud providers adhere to industry standards for data privacy, which means that sensitive data remains protected while undergoing machine learning processing.
Key Machine Learning and Cloud Services for Data Processing
Here are some of the few famous specialized cloud platforms combining machine learning with cloud-based data processing services:
Amazon Web Services (AWS):This consists of several services such as Sage Maker in which the data scientists can scale up the building, training, and deploying of a machine learning model. Additionally, AWS Glue is a managed ETL service that can be enhanced using machine learning in handling data transformation jobs.
Google Cloud Platform (GCP): GCP’s BigQuery ML enables users to create machine learning models directly in BigQuery, making it easier to integrate ML with large-scale data warehouses. TensorFlow, Google’s popular ML library, integrates seamlessly with GCP for model development and deployment.
It provides an end-to-end setting of developing and deploying cloud-based models, thereby making its services with Azure Synapse Analytics and Azure Data bricks superb for processing big data while ML algorithms provide profound insights from the large volume of data.
Applications of Machine Learning in Cloud-Based Data Processing
Machine Learning in cloud-based data processing applies to any kind of industry and, therefore, yields much more accurate and timely insights for each one of them. Here are some of the most notable applications:
Retail and E-commerce
The use of Machine Learning in the cloud helps retailers process customer data and predict buying patterns. This gives retailers a chance to have very personalized recommendations, improve inventory management, and engage in targeted marketing. Processing large datasets in real time allows retailers to adjust their stock, anticipate demand, and better their customers’ experiences.
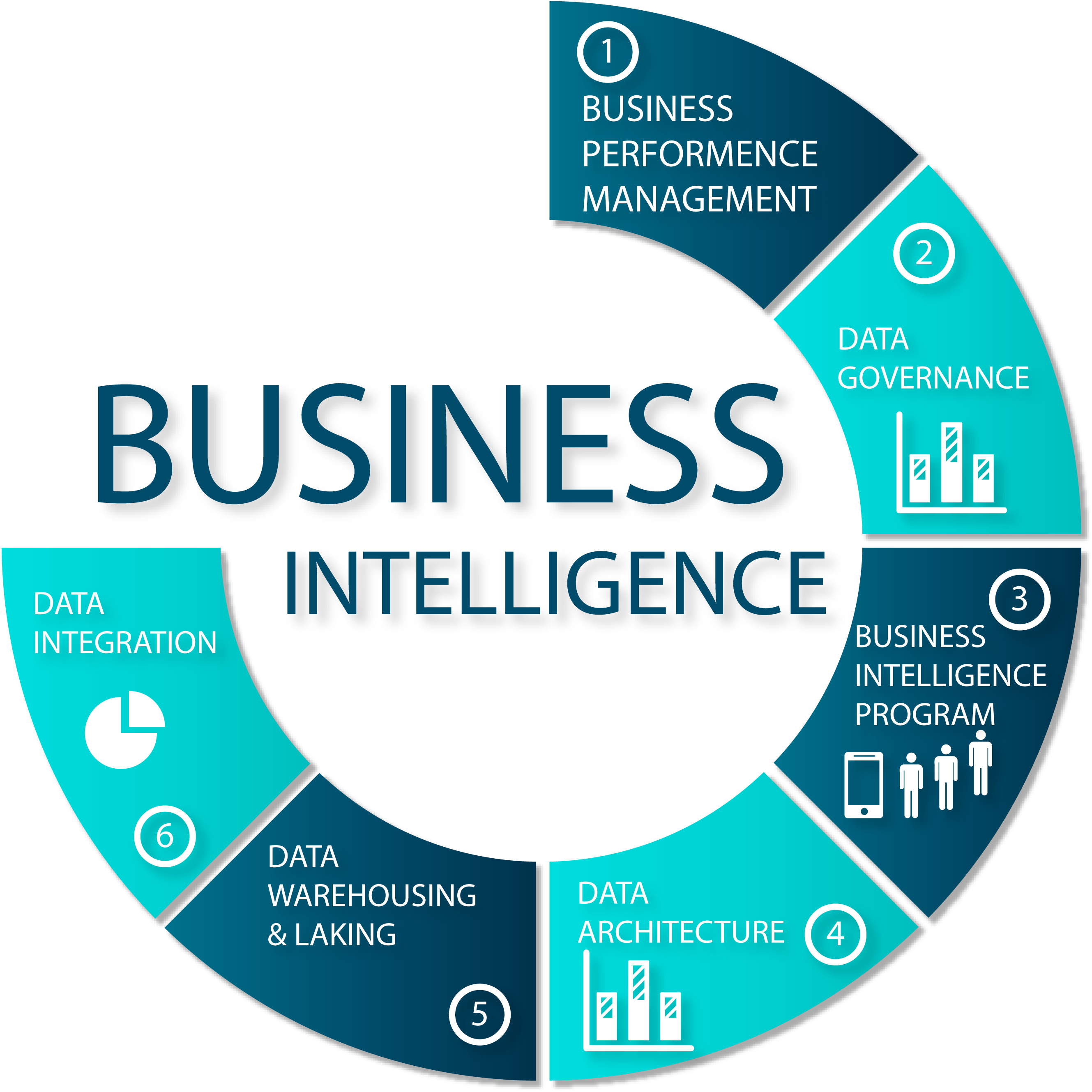
Healthcare
Cloud-based ML models in healthcare processes immense amounts of patient data ranging from EHRs to medical imaging. It allows for a higher accuracy rate in diagnoses, personalization of treatment plans, and prediction analytics on patient outcomes. Cloud solutions also make easier sharing and analysis of medical data securely across institutions.
Finance:
The banks and other financial institutions use Data Analysis in the cloud for fraud detection, credit scoring, and personalized banking services. Since the data are processed in real-time, ML models can identify anomalies, and hence flagging potential fraud before it reaches the customer. Predictive analytics will also make it possible to give better lending decisions due to accurate credit assessments.
Manufacturing
Manufacturers employ cloud-based machine learning for predictive maintenance solutions in order to steer clear of unplanned downtimes. The machine learning algorithms forecast when the machine or sensors need to be serviced in order to curtail expenditure and lengthen the lives of the tools.
Telecommunications:
Telecom companies use cloud-based machine learning to analyze call data records, customer behavior data, and network performance data in order to predict churn, optimize the use of the network, and personalize offers and service.
Advantages of Implementing Machine Learning with Cloud-Based Data Processing
Implementing machine learning into cloud-based data processing offers the following key advantages:
Cost Efficiency: The cloud-based solutions do not require the usage of any physical appliances and allow for the consumption of resources purely based on usage. This means one can easily scale up a machine learning model deployed on the cloud with demand while keeping costs in check.
Accessibility and Collaboration: Cloud solutions make it easy to access data and machine learning models across teams, helping to promote collaboration and make decisions faster. Data scientists, analysts, and business users can work from anywhere and always connect to the same data sources and tools.
Rapid Deployment: Cloud-based machine learning models can be rapidly deployed and allow businesses to respond to conditions in near real-time. Rapid deployment ensures that the insights are actionable and thus drive immediate value.
Data Integration: Cloud platforms can easily integrate data from numerous sources, allowing machine learning models to analyze comprehensive datasets. This holistic view of data improves model accuracy, and insights are relevant to business goals.
Better Security: The major cloud providers have far better security measures, at times much more comprehensive than what a small company would be able to set up on its own. With cloud-based machine learning, businesses can enjoy best-in-class security practices in encryption, identity management, and regulatory compliance.
Challenges and Considerations in Using Machine Learning in Cloud Solutions
There are various benefits of using machine learning in cloud solutions, but challenges and considerations also prevail.
Data privacy and compliance: Cloud storage and processing raise concerns about the privacy of data, especially when industries deal with sensitive information. In this case, organizations have to ensure that cloud providers adhere to regulations such as GDPR and HIPAA as well as maintain robust data governance practices.
Data Transfer Costs: The uploading and downloading of huge amounts of data into the cloud are very costly. Transfer fees should be accounted for, and edge computing or hybrid cloud options considered in order to minimize cost.
Model Monitoring and Maintenance: Machine learning models are only as good as their continuous monitoring and updates in real time. Organizations can look to cloud providers to maintain model monitoring tools but will need to dedicate appropriate resources to this task.
Talent and Skills Gap: Cloud-based machine learning relies on skilled data scientists and ML engineers, who are quite difficult to fill. A gap in the talent market can be bridged by providing training and adopting user-friendly cloud services.
Best Practices for Implementing Machine Learning in Cloud Data Processing
To maximize the benefits of machine learning in cloud-based data processing, organizations need to follow the best practices below:
Choose the Right Cloud Provider and Tools: The choice of appropriate cloud provider should be done based on ML capacity, scalability, and other structural costs which support a well-suited model fit of your business needs.
Investment in Data Quality: “Good quality” in general, is the core reason to have accurate models through perfect predictions. Develop some policies of Data Governance with explicit consistencies, accuracies, and completeness of the information.
Use AutoML for faster deployment: AutoML tools can simplify the process of model development, so even less ML-experienced teams can move forward more quickly to deploy Generative AI models.
Security and Compliance: Work with your cloud provider to ensure that you comply with all the data privacy and security requirements. These include encryption, access controls, and industry standards compliance.
Scalability and Flexibility: Ensure scalability so it goes up or down with your data, and scales with changing demand. Server less functions or containerization can help make deployments scalable or you start small and scale.
Conclusion
Machine learning in cloud solutions has transformed the process of data processing as this facilitates businesses to analyze huge sets of data in real time and make predictive decisions along with automating complex workflows. With a cloud-based approach to ML, cost-effective and scalable solutions for the organization can drive innovation and competitive advantage. Attending to data quality, regulatory compliance, and maintenance of models is a matter that needs to be undertaken before embracing this technology. Nevertheless, the rewards lie in efficiency, reduced costs, and better insights-a strong investment for future-ready business.
You can explore our other blogs
Generative AI, Power BI , Power Business Intelligence
10-31-2024